[Ed. note: Almost exactly a year ago, I started a series of weekly bibliomancy forecasts complete with tarot card and chune of the week. I had planned to complete the tarot deck and then draw the series to a close and reconsider whether to continue the project with a new deck, or in a new way, etc. etc. But then my house burned down, and that deck is gone.
Since then, I have been gifted such beautiful tarot decks, it kind of blows my mind. Thank you!!! My god!!! I do plan on resuming the series—it happens to be a very nice container for me to tell you a little story about the scariest thing that ever happened to me or present a taxonomy of my favorite seasons. But I am going to take a brief break before beginning that new series, because it feels like a moment of silent respect is owed to the small spells tarot deck that took us all the way from the 6 of pentacles to Death.
So, it’ll be back soon. Soon-ish. At the time that feels right. But until then, I’m going to go through my drafts and share some things that have been developing slowly in the background.]
A while ago, while we were driving to the movie theater in the rain, J discovered that Alice Coltrane’s 1971 Carnegie Hall concert was being issued as an LP on Impulse the following month. Neither of us knew. It had never been released in full; significantly, both Pharaoh Sanders and Archie Shepp play on it. I forget how J discovered this news; I think he was searching for the personnel on one of the records (we were listening to Journey in Satchitananda at the time). But one of the first thoughts I had, as J played the first single that had been released, was: Why has no one told us about this?! A form of petulance I can only explain as the result of a digital environment that allows me to remain passive as it informs me that Alvvays or Protomartyr is playing a show nearby soon, and here’s a new Lana single, and you might also like: zzzz.
I was surprised by my own sense of entitlement. I really felt that somebody should have told me about this Alice Coltrane record.
The danger of algorithmic discovery is the illusion of completeness—that everything discoverable is part of the Spotify library, or Google’s search results. That when you ask it a question, the internet shows you all of the potential answers, and not just the ones it expects you to like. (More ominous: the ones it wants you to like.) It’s much more tempting to form an opinion when you think you’ve adequately generalized the field of information, or to treat such opinions as inevitable and factlike.
There’s a certain book of poetry by a well-known and very online writer that always shows up in my search results lately. Its inclusion isn’t always off the mark; it is frequently somewhat relevant to the kind of things I’m looking for. (And to be fair, I actually already own this book, and like it!) But I’m becoming annoyed by the way it shows up over and over, completely irrelevant to what I’m trying to find. Searching for Escher Goedel Bach? How about this book of poetry? Searching for “abject theory”? How about this book of poetry? Angela Carter? Tessa Hadley? Borges’ Labyrinths? I know you’re looking for a novel or a book of theory but have you considered … . If I could press a button that said: I already own this book, leave me alone for fuck’s sake, I would.
//
The world is callow and boring as a result of crippling, massive risk aversion. Risk aversion has increased silently, like a poisonous gas, alongside the pretend certainty of algorithms and big data. Data-driven algorithms offer an attractive fantasy: The idea that things can be predicted with enough data. That a book can be written without risk. The idea that data can meaningfully capture reality at all is a logical fallacy in itself, one we’ve been conditioned to accept because it’s better than uncertainty.
//
“Lost person behavior” describes a methodology for finding people who are lost in the wilderness. Essentially, it means that you consider a person’s disposition and habitual thought when you look for them.
“Looking everywhere” is figure of speech, not an actual option for a search-and-rescue mission, so it is important for rescuers to search first in the most likely places. If a hiker goes missing during a blizzard, there is no time to methodically search a grid from A1 to Z29. If you want to save their life, you have to check the relevant territory of the map to find them. In other words, start looking near the trail, rather than in the remotest corners. But often, lost people are not lost in places where it would be convenient to find them. At some point, the rescuers have to come up with a strategy for searching the inconvenient places, and the premise of “lost person behavior” is that you can further narrow the search parameters by taking behavior patterns into account.
Getting lost is a series of decisions—at least some of which are bad decisions, obviously, but decisions nevertheless. And there are observable trends in what kind of decisions hikers make. For example, inexperienced hikers often get lost trying to cut through a switchback on the trail because they underestimate how difficult the terrain is.
In contrast, experienced hikers tend to seek out notable terrain features once they realize they are lost—they follow water or powerlines, for example. Their wayfinding experience and understanding of topography makes them more likely to follow geographical features which they know will lead to civilization. And, being generally in good shape, they’re more likely to climb a hill in hopes of getting their bearings, while less seasoned hikers will tend to avoid unnecessary physical effort.
Children, once they realize they’re lost, tend to seek shelters like ranger cabins. They still believe that houses have people in them, and that people will help.
//
The basic premise of artificial intelligence, as far as I can tell, is that it is capable of synthesizing so much information that it can become humanlike in its capacity to do anything. If you believe that the brain is basically a hypersophisticated computer, then you only have to build a hypersophisticated computer in order to replicate the intelligence that resides in the brain.
The basic premise of algorithmic social media, as far as I can tell, is that it is capable of synthesizing so much information about your interests, habits, and social affinities that it can become godlike in its capacity to predict what you will react to. And once it has your attention, it will try to sell you a squiggly candle, or some yassified olive oil.
The unquestioned supremacy and usefulness of big data is necessary for both systems to function. Data, as long as you have enough of it, will tell you everything, right? If everything a person does is some form of decision—however subconscious—then theoretically, a high enough yield of decision data should make it possible to predict her every move.
Right?
//
If A.I. is really, really, really super smart and awesome, why isn’t it making me a killing scalping QQQ 0.00%↑ in the stock market?
Surely a hundred years’ market data and history would be enough information to train an AI to flawlessly execute profitable trades, even for retail investors. Right? I know quant investing is a thing, and quite possibly financial institutions are doing everything they can to silo the sort of AI applications that would do away with their edge, but still. It seems odd to me that, out of all the things that we could ask a generative AI to hypothetically do, we have asked it to write term papers and emails and texts setting boundaries with friends.
//
When I was a contract (read: second-class) worker at Google, my team always had ideas to improve the workflow and output of our projects. We didn’t have much of an agenda, except to make our own work less futile and more enjoyable. But whenever we approached our Google overlords with suggestions, we were told that they wouldn’t consider our ideas unless we could somehow quantify the problem and the solution. Changes simply wouldn’t be considered unless they could be demonstrated as beneficial in some sort of measurable, numerical form.
None of us ever pursued this because we had no idea how to go about quantifying our hunches, and no tools to do so. It would have taken a lot of effort to figure out how to quantify and legitimize our subjective hunches. (Of course, we could have generated data by experimenting with different approaches to our work, but somehow, that type of quantification was deemed worthless.) It was implied that we were not being paid to solve problems, but just to do what we were told.
//
It’s like that old joke: A cop comes across a drunk searching for his keys in the light of a streetlamp. The cop helps the man search, and after they’ve scoured the area, the cop says, “Hey, buddy, are you sure you lost your keys here?”
The drunk says, “Oh, no. I lost them over there, in the park.”
The cop is dumbfounded. “If you lost them in the park, why are you looking for them on the street?”
“This is where the light is,” the drunk replies.
//
I have many friends who are more open-minded about A.I. than I am, finding ways to use it in their projects, always with intelligence and nuance. I agree that any tool can be used in good ways and bad ways. But I think my objection to AI has to do with a suspicion that it contains a massive thought error, a huge structural weakness, in that it begins from the idea that its originary data set is complete enough to be useful.
I have worked at enough tech companies to realize why they refuse to consider any form of action that had not been quantified: Because if data has been quantified, it’s less risky. Or theoretically less risky—you could use the streetlamp fallacy to “prove” that more keys are found in the light than in the dark, and propose that the most cost-effective measure would be to search in the easiest places. You would never find the lost keys, but oh well! If someone ever called you to account for your actions, at least you would have data to hide behind.
Beyond that, it seems incredibly foolhardy to assume that everything worthwhile and meaningful that goes into a piece of writing is quantifiable. For the purposes of generative A.I., the only useful information about the world is quantifiable information. And in collecting quantifiable information, we will always be at the mercy of our instruments, only able to see at the depth and granularity of our measuring devices.
The world that exists is beyond the world that we can measure. The territory is vaster than the map. Data materialism seems to be the underlying premise of AI—the idea that the essential nature of things can be captured if you just have enough 1’s and 0’s. This seems both arrogant and sort of stupid to me. And totally wishful. The basic unit of consciousness is the moment—this one, which is also the only one. The moment, with its ragged edges, with its liquid center, with its impossible arrivals and departures, the only place where beauty lives, the stream of images, the live wire in a conversation for that moment before someone steps across a threshhold and says something they can’t take back.
//
In “Why A.I. Isn’t Going To Make Art,” Ted Chiang posits that art is discernible from other things in that it is produced by an accumulation of hundreds upon thousands of choices. Choices—not just boolean logic derived from vast pools of data.
But I would push this idea further. Art isn’t just about making choices. It’s about taking risks. And risk is complex because it’s so relational and contextual. It wouldn’t be much of a risk to say today that the Rangers are going to win the 2023 World Series. They did. It is known. It wouldn’t be much of a risk to say that children should not be painting radium watchfaces in factories. It wouldn’t be much of a risk to write a novel about two Irish students in an on-again, off-again love affair marred by frictions of class and problematic desire because that novel has already been written, and did great numbers.
We all have our own metrics for art, and I’ll admit that I favor risk. I will always respect a moment of risk, even if I don’t love its aesthetics or outcome. As a result, my assessments will differ from those who like their art to feel recognizable or comforting. But there is always some element of risk in good art, and unless we end up massively changing our categories of aliveness, A.I. will never be able to take a risk. Not one, not ever.
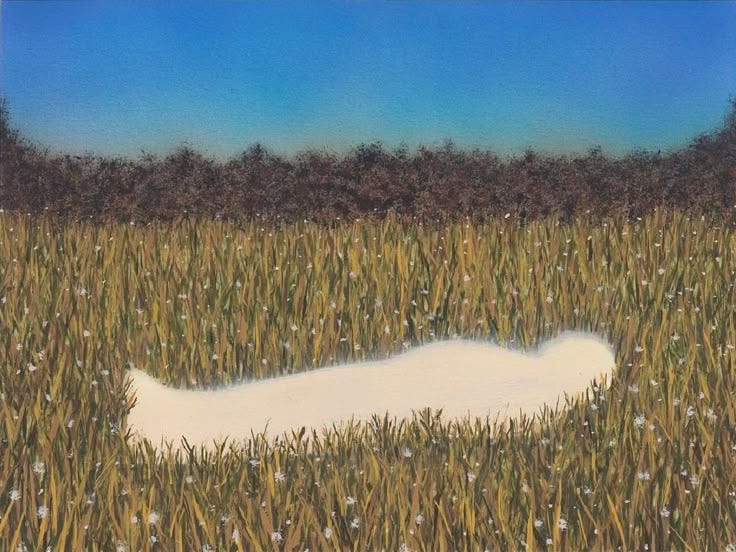
//
I took a long walk in the Shops at Santa Anita during our autumn heatwave in LA, and over the course of my 10,000 steps, I got quite well-accquanited with the Looks for Fall as represented on the front-facing mannequins.
Here it is, get ready:
Black and white. And navy.
One particularly chilling display (H&M, I think) showed four male mannequins wearing barely differentiated all-navy looks. Navy blazer and navy slacks. Navy coach’s jacket and navy slacks. Navy nehru collar and navy slacks.
Various corners of our cultural commentariat have been lamenting the same-ness and stuck-ness of our time. It’s all Marvel movies, rebooted Beetlejuice, rebooted Twister. All the girls have the same pillowy filler-face. Everybody is wearing beige Xanax sweats. All the new literary novels have the same bold color/abstract blot cover (unless they all have the same gradient cover, which I wrote about TKhere.) The world changes direction like a massive school of fish. Words that people use to avoid TikTok filters suddenly appear in real life. Unalived. Graped. SA’d. So many this. The way I. The woman you are. Demure. (The fact that people can unashamedly use words that have been in everyone’s mouth like this honestly freaks me out. I find it fucking repulsive.)
When I saw those H&M mannequins, I thought: I know why it’s all the same. It’s camouflage.
It’s because we’re all hiding.
Lost person behavior is the premise of algorithmic social media. Our algorithms know how we behave, what we fear, the decisions we’ve made. And they can follow us relentlessly, selling us serums and showing us articles that will make us so mad that we have to share them with others or have a public meltdown.
Data has risen off of us in huge, stinking clouds. All those BuzzFeed “Which Hogwarts house are you?” quizzes. The fact that you always buy an onigiri when you’re stresed out about something. You clicked on an article about attachment styles once, three years ago? Just throw your phone away now. Spotify knows how many times you listened to “Murder on the Dancefloor” this year. (An unhinged number of times.) At first we were too naive to know how much we were giving of ourselves. And by the time we realized our mistake, we had become so habituated to the long, chilly tethers that keep us hypothetically connected to old friends and the ecosystem of Facebook-dependent app logins that it seems too difficult to extricate ourselves.
Advertisers can hunt us down and sell us things that we aren’t even aware that we wanted. Having issues with facial redness? the Instagram ad whispers to me. Well, gosh, now I am.
//
I hate it when someone goes to great length to point out everything that’s wrong without also indicating the new way to move. So I won’t play around. We need risk.
Of course, this is still a vague proclamation—because risk is situational and personal, you are the only one who will ever know the difference between a dangerous-looking thing that feels safe to you (reading poems in a crowd? Baby I can read poems in the supermarket) and a safe-seeming thing that feels dangerous (telling someone I’m mad at them—always my last resort). Your risks are real because they feel real. Your greatests risks might be invisible. They demand that you get lost. And in the future, being willing to be lost will be the most valuable quality any of us can cultivate.
To be continued ….
//
Idk, what do you think? I’ve been working on this for so long I don’t even know. I have a habit of writing essays that are actually books and books that are actually black holes. But whatever it is, I feel it.